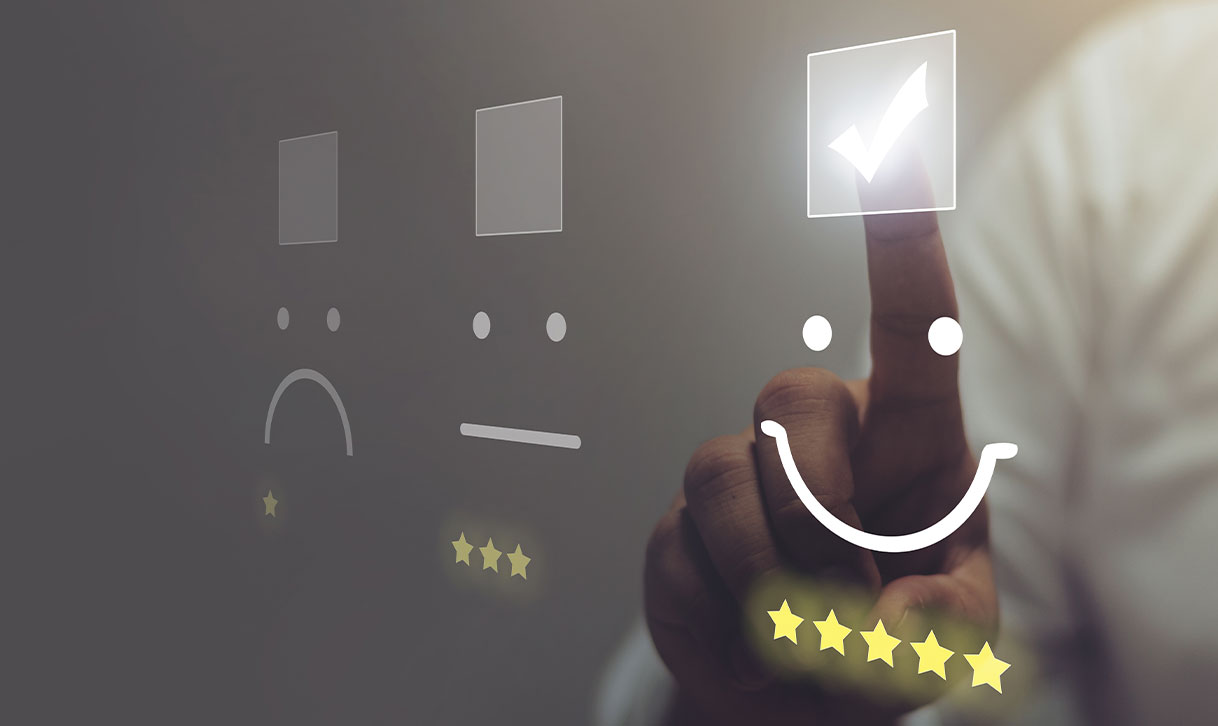
How to Improve Multilingual Speech Recognition Performance? In View of Acoustic Modeling
Date : 2021-10-27 View : 1728
As the development of modern technology, cross-culture communication become more frequent and code-mixing becomes a common phenomenon. People are getting used to mingle different languages into a single sentence, sometimes even intuitively.
The code-mixing phenomenon brings much challenges to the automatic speech recognition system development. How to develop a reliable multilingual speech recognition system have become a heated topic within the industry.
Recognition of the embedded language is the pressure point. This means researchers must deal with two problems. The first one comes from recognition of the matrix language accent in embedded language. The second one is how to balance the cost and effectiveness in developing the multilingual speech recognition model, especially when the embedded language comes from a data-scare language.
There are two main directions we may take into consideration in terms of acoustics modeling: applying multilingual datasets in training data deployment and applying transfer learning.
We will use an example of developing a Mandarin-English bilingual speech recognition system for real world music retrieval in this passage to expand the idea.
Multilingual datasets training data for acoustic modeling
In training data deployment, in addition to Chinese dataset and English dataset, Chinese-English code-mixing dataset is recommended to train the ASR model, as researches shows that compared with using monolingual Chinese and English training dataset, using Chinese-English speech data for phoneme clustering and model training, the error rate of the baseline model in Chinese-English mixed speech recognition is reduced by 37.93%.
Global phone set lexicon
Compared with building and training an acoustic model from 0, transfer learning can quickly achieve a favorable outcome without costing large amount of time and resources. Using transfer learning for reference, we can adopt a global phone set lexicon in building an adaptation acoustic model, reducing the amount of embedded language data as required for training while lowering word error rate.
For more data insight, contact our data experts (business@magicdatatech.com).