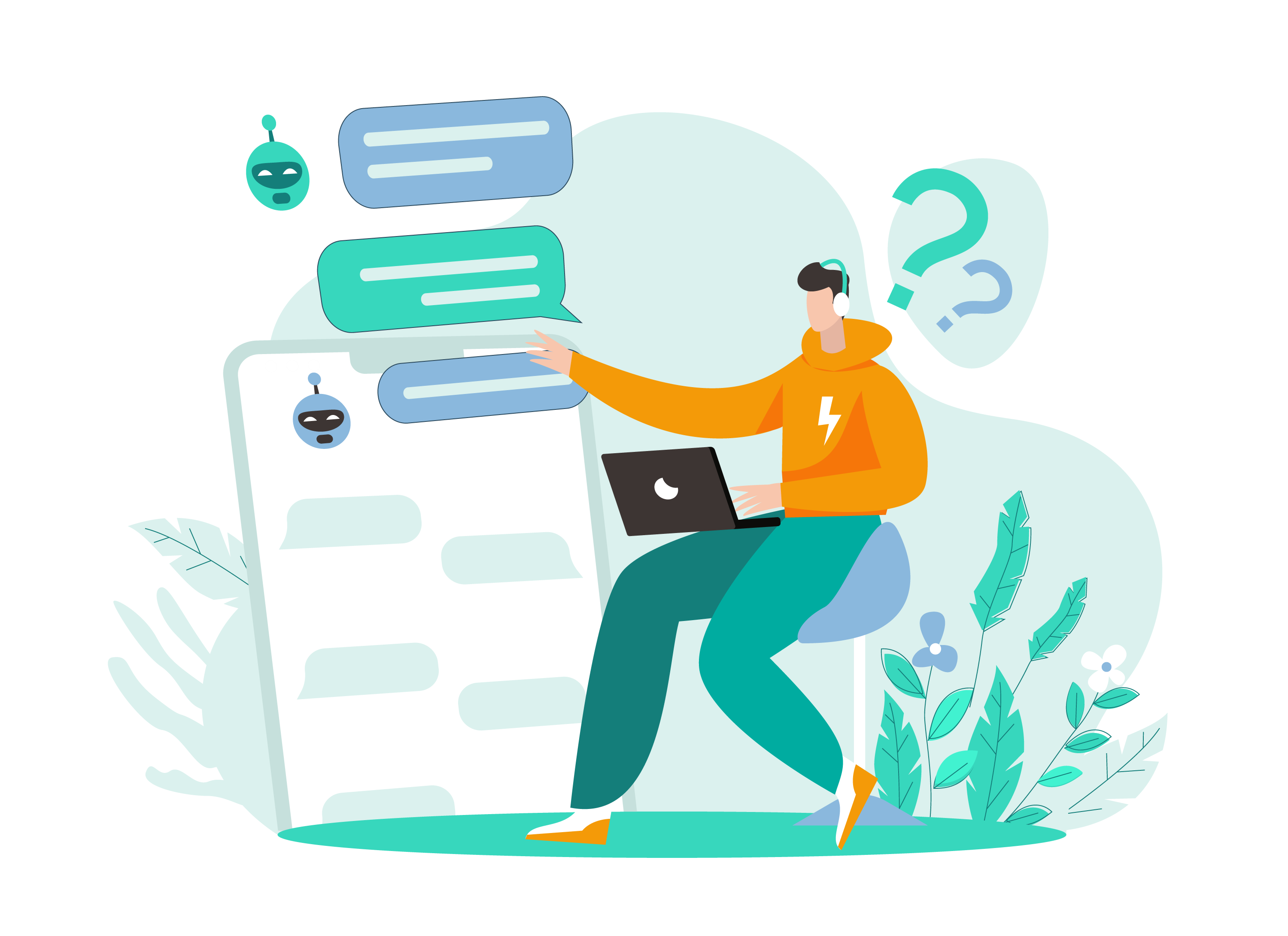
Do You Think the Sweet Customer Service Lady is Real
Date : 2022-06-08 View : 1003
Whether it is pre-sales or after-sales, customer service is the bridge of communication between merchants and customers. No one can resist a customer service lady with fluent and sweet voice, good logical thinking ability and communication skills. But is every customer service a beautiful young lady or a handsome young man? Naturally not.
With the development of artificial intelligence, intelligent customer service continues to replace or assist human customer service in all walks of life. Among them, conversational AI has become an important position for enterprise intelligent customer service. According to data from iResearch, the market size of domestic conversational AI in 2021 will be 4.5 billion yuan, driving a scale of 12.6 billion yuan. It is estimated that in 2026, the market size of conversational AI will reach 10.8 billion yuan, and the driving scale will exceed 38.5 billion yuan.
Through intelligent customer service, most simple problems can be quickly solved by self-help, and complex problems have the opportunity to be solved manually and efficiently. In the process of serving users, intelligent customer service can provide core capabilities of intelligent customer service such as question recommendation, question understanding, dialogue management, answer supply, speech recommendation and conversation summary, in order to achieve low-cost, high-efficiency, high-quality communication with users.
Although the proportion of intelligent customer service in the market exceeds that of manual customer service, the humanized intelligent customer service experience still faces many challenges due to the technical development of voice recognition, speech synthesis, intelligent dialogue, knowledge graph and other fields.
Challenge
1. Domain adaptation of the vertical domain model
In the field of intelligent customer service, dialogue is a key link in service. Due to the different professional vocabulary in each field, general speech recognition models or natural language processing models cannot always accurately identify the true meaning behind each keyword. Since the academic community currently uses general-purpose deep learning models trained on open source clean data, model adaptation for each domain is the key to deploying intelligent customer service in each vertical domain. For example, customer service in the medical field and customer service in the field of financial lending sales are definitely different. The construction of this question and answer database requires long-term accumulation and manual collection and sorting.
2. Real-time feedback requirements
Studies have shown that human tolerance for smartphone response time is about 4 seconds. In normal chat, the time tolerance for interaction between people is probably no more than 5 seconds. This shows that intelligent customer service needs to have the ability to deal with problems in real time, that is, online reasoning of knowledge graphs, online recognition and synthesis of speech, understand the user's intent within the interaction tolerance time and give the user a satisfactory response. This requires high real-time performance of each module of the intelligent customer service system.
3. An anthropomorphic user experience
At present, the intelligent customer service on the market is still in the early stage of development. It can only retrieve keywords from the inherent knowledge database to answer questions, and cannot intelligently generate a question-and-answer mechanism. A major challenge is how to mine a sound knowledge graph from the data of each vertical domain and guide the intelligent customer service to generate a sound question and answer generation mechanism, so as to face various problems more tactfully. Often, instead of asking any questions, they all answer with the same content, causing various complaints from customers. Furthermore, there are high requirements for the synthesis of simulated human voices in the field of speech synthesis. If the customer finds that a robot is the first to answer their call instead of a human customer service agent, they often feel annoyed and frustrated. Figuring out how to synthesize speech that is warm, logical, and able to understand user intentions like a human and give reasonable solutions is also one of the keys to the popularization of intelligent customer service.
Solution
1. Collection of professional data
Adaptation to different domains is the key to distinguishing humans from intelligent machines. Facing the promotion of intelligent customer service in each vertical domain, it is naturally inseparable from the collection of various intra-domain data. Adapting a deep model to this field through a small amount of intra-domain data is an eternal topic in academia and industry. The key to this topic is the collection of data in the domain. Data collection involves a lot of professional knowledge and consumes a lot of manpower and material resources. Professional data companies like MagicData are needed to provide the cornerstone for artificial intelligence. At present, MagicData has a large number of customer service dialogue data, involving many different fields, scenarios, and languages. Some examples are as follows:
MDT-NLP-F022 English Medical Customer Service Text Corpus
MDT-NLP-F021 Chinese Educational Customer Service Text Corpus
2. Online training and lifelong learning
Whether it is the real-time feedback mentioned above or the anthropomorphic user experience, intelligent customer service needs to be smarter and smarter; able to quickly understand customer intentions and know the solutions well. This relies on optimizing the service process, improving the customer service experience, accumulating online data, training data online and letting the deep learning model learn for life. Setting up online data accumulation, online learning, and lifelong learning can build a more complete knowledge map, making customer service more intelligent, witty, and responsive.
Concluding Remarks
The key to the popularization of intelligent customer service and the improvement of user experience is a large amount of intra-domain data. Just like the concept of Data-Centric AI proposed by NIPs in 2021, companies from diverse industries such as automotive, electronics, and medical device production have achieved significant gains in deploying AI and deep learning-based solutions in manufacturing scenarios compared to traditional rule-based implementations. progress. Experiments also show that data-centric improvements can build computer vision applications 10 times faster, reduce application deployment time by 65%, and improve accuracy by up to 40%. Data usage is the cornerstone of AI development.