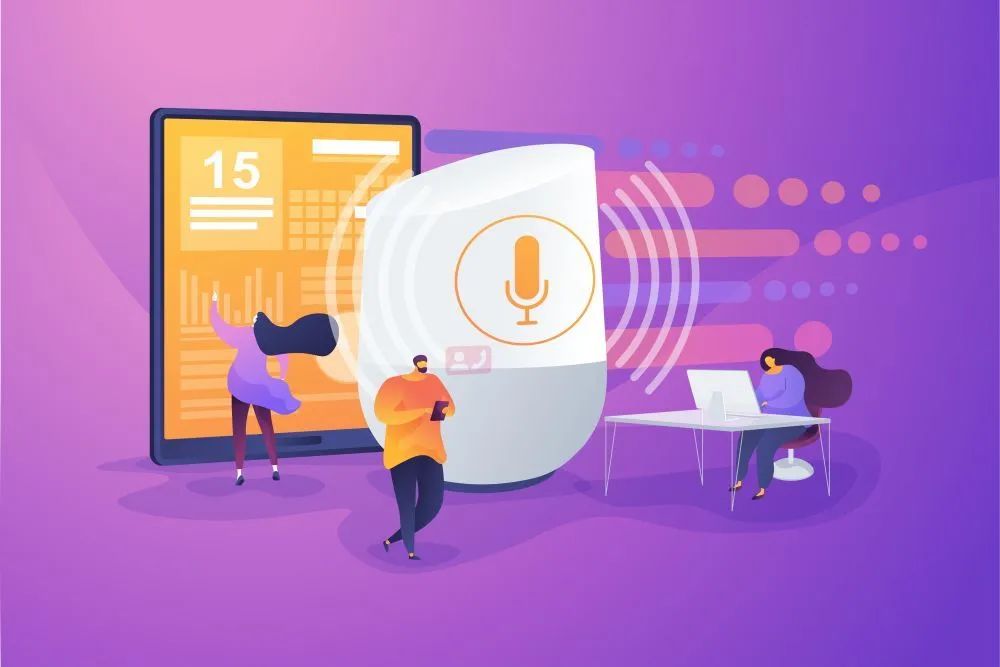
Environmental Adaptation of Voice Assistants
Date : 2022-07-15 View : 1273
Voice assistants have been playing a more and more important role in our life. However, sometimes a smart voice assistant indoors may become "dumb" when it is used in outdoor environment. Just like us humans, the voice assistant gets panic when it arrives in a new environment. People tend to be more nervous and cautious when communicating in a non-native environment. Humans are uncomfortable with things they are not familiar, not to mention artificial intelligence algorithms. The voice assistant need to adapt to different domain, which is called transfer learning, an important but difficult problem in speech recognition, speech synthesis, speaker recognition and other speech fields.
The artificial intelligence algorithm inside the voice assistant is often learned from a large amount of data. This large amount of data cannot cover all application scenarios, which leads to some new scenarios, such as curved conference halls, open squares and other scenarios. The accuracy of speech recognition is greatly reduced. Or, models trained on a large number of voice dialogue data recorded in recording studios cannot be directly used in some professional fields, such as e-commerce customer service, financial intelligent customer service, and intelligent medical fields. Due to the lack of domain knowledge, the model effect is not satisfactory in new scenarios. How to adapt the model to each vertical domain is generally considered from two aspects.
Transfer Learning Algorithms
Transfer learning refers to the model we train on data of one scenario, which can adapt to the transfer learning algorithm, apply this model to other scenarios, and try to keep the performance of this model from being affected by changes in the environmental domain as much as possible. Transfer learning relaxes the assumption that the training data must be independent and identically distributed (i.i.d) with the test data, motivating us to use transfer learning to solve the problem of insufficient training data. In transfer learning, training data and test data do not need to be i.i.d. Models in the target domain do not need to be trained from scratch, which can significantly reduce the need for training data and training time in the target domain. According to the summary of S. J. Pan and Q. Yang, A survey on transfer learning, transfer learning algorithms can be divided into the following categories according to different situations:
Although there are many transfer learning algorithms mentioned above, the execution of the algorithm is still inseparable from the support of the data in the domain. Almost none of the above algorithms can be implemented without in-domain data.
Intra-domain data adaptation
The simplest and most effective transfer learning method is to fine-tune an existing model with a small amount of in-domain data. Make the existing model adapt to the current data scene. The above transfer learning algorithms are also inseparable from the support of data in the domain. For voice assistants to be applied in various vertical domains, it is inseparable from learning on the data of each vertical domain. This requires a professional data company team like Magic Data to provide vertical data for many researchers in industry and universities to support the research of the above-mentioned transfer learning algorithm and the application of voice assistants in various fields. Magic Data is a leading global AI data solutions provider, with over 400 speech datasets in more than 60 languages and dialects covering a great variety of scenarios. Examples of which are as follows:
MDT-ASR-E026 Mandarin Chinese Conversational Telephone Speech Corpus
MDT-ASR-E059 Turkish In-Vehicle Scripted Speech Corpus—Smart Mobility
For more information, check out www.magicdatatech.com/datasets