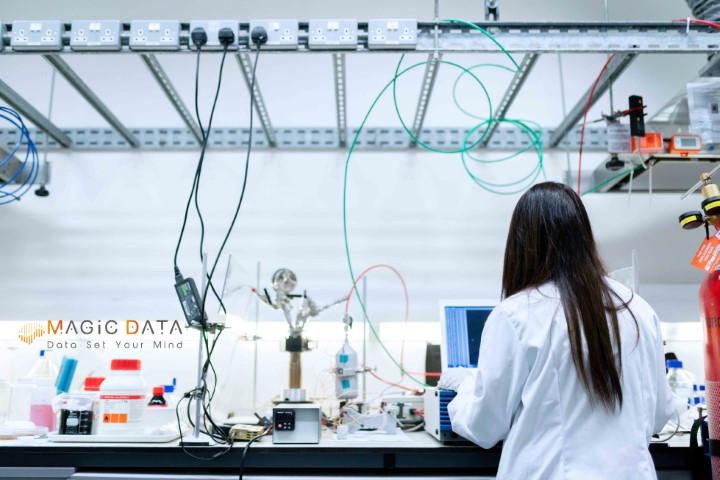
How to Start Your Machine Learning Projects with MagicData-RAMC?
Date : 2022-04-26 View : 1598
As a collection of high quality and richly annotated training data, MagicData-RAMC (free download available at magichub.com) is applicable to a series of research. This article will introduce 3 experiments related to speech recognition, speaker diarization and keyword search based on MagicData-RAMC conducted by Magic Data, together with the Institute of Acoustics, Chinese Academy of Sciences, Shanghai Jiao Tong University and Northwestern Polytechnic University.
1. Automatic Speech Recognition
The research team uses a Conformer-based end-to-end (E2E) model implemented by ESPnet2 toolkit to conduct speech recognition. The Conformer model is composed of a Conformer encoder proposed in and a Transformer decoder. The team adopts 14 Conformer blocks in the encoder and 6 Transformer blocks in the decoder. Connectionist temporal classification (CTC) is employed on top of the encoder as an auxiliary task to perform joint CTC-attention (CTC/Att) training and decoding within the multi-task learning framework. During the beam search decoding, the beam size is set to 10.
The research team computes 80-dimensional logarithmic filterbank features from the raw waveform and utilize utterance-wise mean variance normalization. The frame length is 25 ms with a stride of 10 ms. SpecAugment is applied with 2 frequency masks and 2 time masks for data augmentation. The maximum widths of each frequency mask and time mask are F = 30 and T = 40 respectively. The input sequence is sub-sampled through a 2D convolutional layer by a factor of 4. The inner dimension of position-wise feed-forward networks in both encoder and decoder is 2048. The research team applies dropout in the encoder layer with a rate of 0.1 and set the label smoothing weight to 0.1 for regularization.
The multi-head attention layer contains 8 heads with 256 attention transformation dimensions. CTC weight used for multi-task training is 0.3. We train the model using the Adam optimizer with β1 = 0.9, β2 = 0.98, ǫ = 10−9, gradient clipping norm 5 and no weight decay. Noam learning rate scheduler is set to 25000 warm-up steps. The maximum trainable epoch is 100. The final model is averaged by the last 5 checkpoints
The training set is made up of two parts: the 150 hours training set of MagicData-RAMC and the 755 hours MAGICDATA Mandarin Chinese Read Speech Corpus (MAGICDATAREAD). The two sets are combined to compose over 900 hours of data for training. The experimental result is shown in terms of character error rate (CER) in Table 1.
Table 1: ASR Results (CER%) on dev and test set
2. Speaker Diarization
For SD task, the baseline system consists of three components: speaker activity detection (SAD), speaker embedding extractor and clustering.
Following Variational Bayes HMM x-vectors (VBx) experiment setting, Kaldi-based SAD module is used for detecting speech activity. The research team adopts ResNet trained on VoxCeleb Dataset (openslr-494), CN-Celeb Corpus (openslr-825) and the split training set of MagicData-RAMC to obtain the speaker embedding extractor.
For training details, the SAD module utilizes a 40-dimensional Mel frequency cepstral coefficient (MFCC) with 25 ms frame length and 10 ms stride as input features to detect the speech activity. ResNet-101 with two fully connected layers is employed to conduct speaker classification task with 64-dimensional filterbank features extracted every 10 ms with 25 ms window, and additive margin softmax is used to get a more distinct decision boundary. The raw waveform is split every 4s (400 dimensions) to form ResNet input. The speaker embedding network is trained by stochastic gradient descent (SGD) optimizer with a 0.9 momentum factor and 0.0001 L2 regularization factor.
Besides, 256-dimension embeddings are conducted dimensionality reduction using probabilistic linear discriminant analysis (PLDA) to 128-dimension. Embeddings are extracted on SAD result every 240 ms, and the chunk length is set to 1.5s. For the clustering part, the research team use Variational Bayes HMM [6] on this task. An agglomerative hierarchical clustering algorithm with VBx is conducted to get the clustering result. In the VBx, the acoustic scaling factor Fa is set to 0.3, and the speaker regularization coefficient is set to 17. The probability of not switching speakers between frames is 0.99. The experimental result is showed in Table 2.
Table 2: Speaker diarization results of VBx system
3. Keyword Search
The research team carry out the KWS task following the DTA Att-E2E-KWS approach proposed in relying on attention-based E2E ASR framework and frame-synchronous phoneme alignments. The KWS system is based on our Conformer-based E2E ASR system described in Sec 3.1. We adopt the dynamic time alignment (DTA) algorithm to connect a frame-wise phoneme classifier’s output posteriors and the label-wise ASR result candidates for generating accurate time alignments and reliable confidence scores of recognized words. Keyword occurrences are retrieved within the N-best hypotheses generated in the joint CTC/Att decoding process.
The keyword list is built by picking 200 words from the dev set and provided together with the dataset. In the DTA Att-E2E-KWS system, the frame-wise phoneme classifier of the KWS system shares 12 Conformer blocks with the E2E ASR encoder while retaining the top 2 Conformer blocks unshared. The classifier outputs posteriors of 61 phonemes, including silence and noise. The KWS system is optimized following the setup in Sec 3.1. During the inference stage, we retrieve keywords within ASR 2-best hypotheses. During KWS scoring, a predicted keyword occurrence is considered correct when there is a 50% time overlap at least between the predicted occurrence and a reference occurrence of the same keyword. The results are shown in Table 3.
Table 3: Results on dev and test set for the Conformer-based DTA Att-E2E-KWS system
We hope this introduction can be of some help for your further studies and MagicData-RAMC can facilitate the applications of various speech-related research.
You are welcome to share any insight or comment to open@magicdatatech.com.