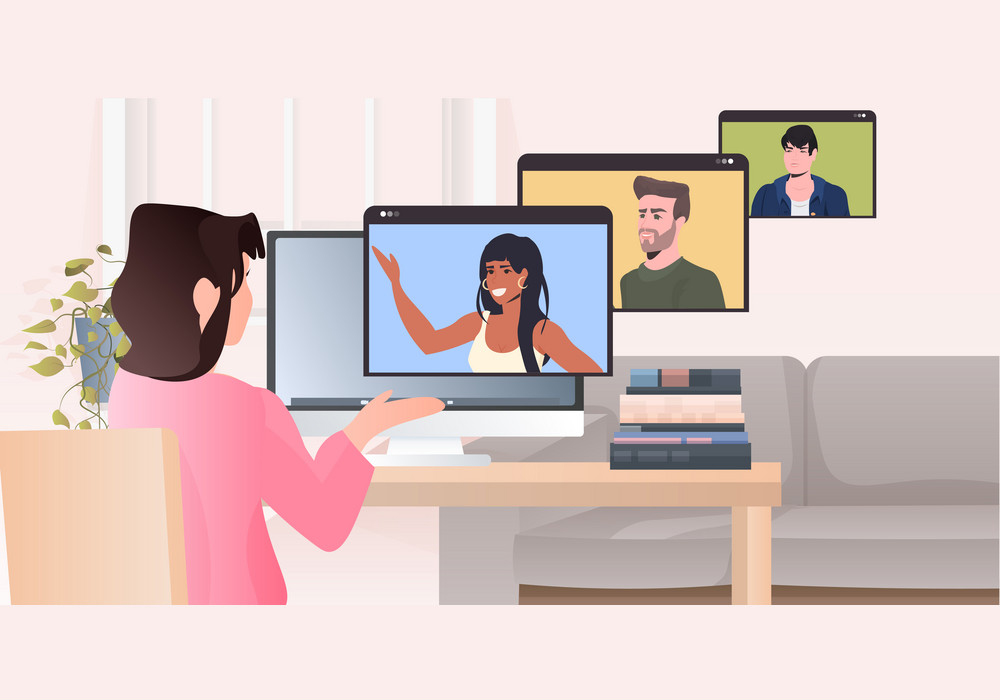
Work/Class from Home—Does Your Online Meeting Assistant Work Well?
Date : 2022-06-01 View : 798
Since the end of 2019, COVID-19 has upended many different parts of our lives. The number of global online office and online teaching staff has increased significantly. Facebook CEO Mark Zuckerberg said during an online employee meeting that Facebook will take 5-10 years to allow half of its employees to permanently work remotely. Zuckerberg said that data from a Facebook employee survey showed that 20% of employees are "very interested" in maintaining full remote work after the epidemic isolation measures are lifted, and another 20% are "somewhat interested" in this option. Working from home has a variety of benefits, including allowing for more work location flexibility. The prevalence of COVID-19 has become a major turning point from face-to-face meeting/working to online office/class. The online conference voice assistant is the largest application of online conference/teaching. Compared with ordinary speech recognition application scenarios, there are more challenges in speech recognition in online conference scenarios.
Challenge
Since online meetings or online teaching and classes are all conducted in the home environment; home noise, equipment diversification, multilingual mixing, network delay and equipment performance can bring a lot to real-time speech recognition and transcription challenges.
1. Interference from background noise of life
When parents work from home, children take online classes at home, and everyone lives in the same house; it is easy to generate noise that interferes with each other. Whether from the same home or the surrounding environment, the background din of multiple speakers can often hinder the ability to communicate during a video or audio conference, or when talking in a car, on a cell phone, or with a digital voice assistant. At the same time, children's voices, language, and often erratic behaviors in families are far more complex than adults. Speech recognition devices need to take into account variables such as children's language patterns, language structure, and intonation (which can vary greatly with age), not to mention issues such as syntax, grammar, and pronunciation.
2. Mixed language switching
Due to the development of globalization, the phenomenon of English words in the Chinese context is often mixed in the actual daily communication of human beings. This is called code-switching in academics, and it is one of the important challenges facing the current speech recognition technology. The main technical difficulties are as follows: the non-native accents formed by the embedded language are seriously affected by the host language, the difference between the phoneme composition of different languages brings great difficulties to the hybrid acoustic modeling, and the labeled hybrid speech training data is extremely scarce.
3. Difficulties of real-time speech transcription
Is it better for students to take online classes and for staff to hold online meetings or is it better to have face-to-face classes or meetings, and everyone can concentrate? Currently, real-time online meeting transcription and meeting outline transcription are the biggest needs of online meetings. Real-time transcription involves not only real-time issues, but also the difficulty of speaker logs and speech separation. These tasks are even more difficult if the discussion is heated, or if there is interference from other people in the background.
Solution
Any task related to speech recognition based on deep learning is inseparable from the support of data. Data is the cornerstone of deep learning, and conference scene data is the cornerstone for solving the challenges related to speech recognition in conference scenes. For speech recognition related tasks in conference scenarios, it can be implemented from the aspects of related scene data recording and multi-task algorithm integration framework.
1. Real meeting scene data and multilingual corpus collection
The decrease in the accuracy of speech recognition caused by the mismatch of domains caused by the corresponding scene problems of deep learning can be attributed to the lack of data. At present, the implementation of data-driven speech recognition in conference scenarios is the most mature and stable solution at present. The data-driven technical route also means higher requirements for data and computing. For example, the results that required thousands of data before may now require tens of thousands of data. However, the collection of large amounts of data will take up a lot of human and financial resources. The main responsibility of an algorithm engineer is to study algorithms, and professional data collection companies should be hired to do such professional tasks. This requires Magic Data to be committed to becoming the world's leading multi-modal data service provider. Committed to high-quality data collection, clear vertical classification, and careful data cleaning services, for conference scenarios. A multilingual and noisy conference scene voice library is ready-to-use. Example of this is as follows:
MDT-ASR-D015 English Conversational Telephone Speech Corpus—Telephony
MDT-ASR-B007-A3 Residential Noise Dataset — Computer
2. Research on multi-task algorithm integration framework
The speech recognition of the intelligent conference scene mainly involves: speaker log, speech separation and separation, speech enhancement and speech recognition module. Most of the current research work of each module is trained separately, and the strategy is integrated when it goes online. Due to individual optimization, there is the problem of local optimality, and it is impossible to achieve the overall optimality of multiple modules, which is the current direction of academia and industry. At the same time, due to real-time requirements, the algorithm model also needs to be smaller and more accurate. However, the research of the algorithm still needs to be based on the existing data. At present, some papers are based on simulated data and do not have the characteristics of real data, and there will still be gaps in the actual implementation. In view of this, our company also provides some conference scenarios and multilingual voice databases for research workers. Examples are as follows:
MDT-ASR-E026 Mandarin Chinese Conversational Telephone Speech Corpus
MDT-NLP-A015 Chinese Financial Meeting Text Corpus
Data is the propellant of development of voice technology in the future. Data determines how competitive products an algorithm engineer can create. Data companies like Magic Data are professional team that builds the cornerstone of data for us.